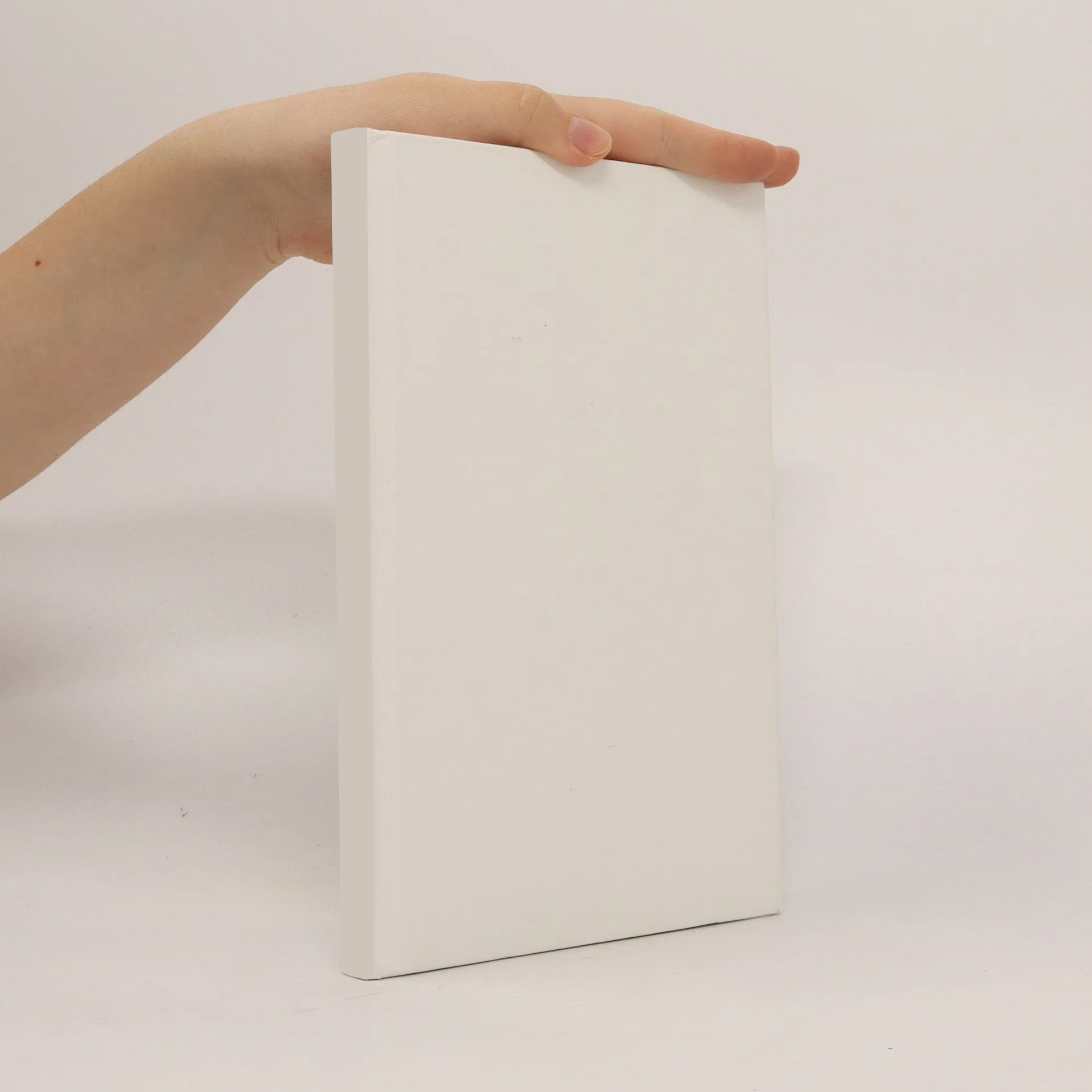
Relational semi-supervised classification using multiple relations
Autoren
Mehr zum Buch
Nowadays new technologies allow to store vast amounts of data, hence the amount of collected data grows steadily. This trend is positive on one hand since more knowledge is available, but on the other hand it may lead to information overload on the side of the user. This means, we need to provide the user with facilities that help organizing the collected data. Therefore usually supervised classification algorithms, under the assumption that data instances are independent and identically-distributed iid, are applied. Meaning that only inherent attributes of the instance itself are taken into account. However, using standard supervised classification methods may lead to less accurate results because of the following three issues: First, the iid assumption may not always hold, i. e. often relations and dependencies among data instances exist, but are ignored, second, if relations are taken into account, often only one is considered even if multiple exist, and third, the required labeled data for supervised classification is scarce and costly to obtain. Examples for data where the iid assumption does not hold are: Web pages connected by hyperlinks and scientific publications which are related by common authors, venue or citations. Apart from text documents, relations can also be observed in other domains like social tagging systems where users are related to each other by sharing the same resources. We also consider situations where a relation is not explicitly given, in these cases a relation can be constructed using similarities. In the medical domain for instance, patients could be connected to other patients if they have similar measurements (time series of blood pressure, heart rate, etc.). In each domain mentioned above, labeled data is scarce while the cost of expert annotation is high, and multiple relations among data instances exist, thus we will address all three issues in this thesis. We propose and analyze several semi-supervised relational algorithms using multiple relations. We investigate their benefits in different domains and show that independent of the type of data or the area of application, semi-supervised relational methods exploiting multiple relations are highly predictive and mostly outperform state-of-the-art algorithms.