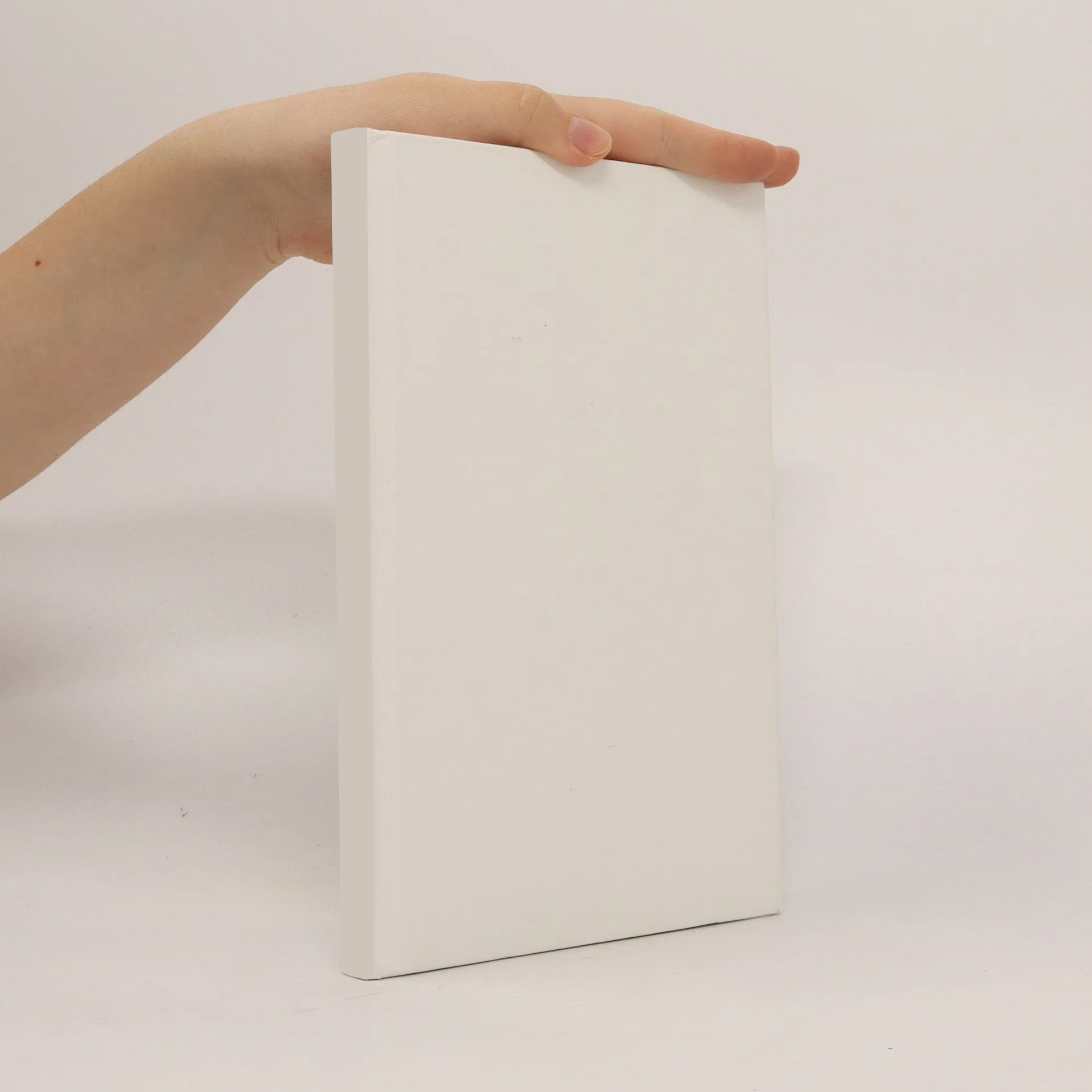
Structural analysis of patterns and shapes using hierarchical vibrations
Autoren
Parameter
Kategorien
Mehr zum Buch
The structural representation of shapes and pattern spaces is an important theme in computer vision and image analysis. For example, shape is one of the main sources of information that can be used for the classification and segmentation of images, as well as for multi-modal data fusion. Image segmentation can be seen as an ill-posed, inverse problem, which may have multiple possible solutions that do not continuously depend on the data. One reason for this is that images often come noisy and incomplete, while the texture and shape of visual objects may vary dramatically due to articulation, occlusion or variations in perspective and lightning conditions. A popular top-down approach to this problem is to collect a manually labelled training set of images from which the shape and texture variation in an object class can be learnt. Probabilistic graphical models require intensive training for separating valid from invalid non-linear variation. This may not always be possible. Further, determining a prior feature distribution that is both, informative and general, is not straightforward. A structural decomposition approach is presented for the model-based analysis of variable visual shapes and patterns in images without the need for training. The finite element shape decomposition principle has been extended to describe and match deformable visual objects at different, spatially resolved levels of abstraction and detail. A set of knowledge-based a-priori constraints in combination with general heuristics effectively condition the model to produce anatomically plausible shapes. The hierarchical shape decomposition approach offers a complete characterisation of the fitted shapes in terms of qualitative and quantitative features. The visual object model has been proven to represent various object categories. The a-priori constrained parametrisation allows interpolation between exemplar configurations, and accounts for “unknown” variations very well. We show experimentally that a valid parametric prior can be built from expert prior knowledge in a straightforward manner. The advantages of the hierarchical approach imply the training of specialised models from an abstract prototype and benefit of categorisation.
Buchkauf
Structural analysis of patterns and shapes using hierarchical vibrations, Karin Engel
- Sprache
- Erscheinungsdatum
- 2011
Lieferung
Zahlungsmethoden
Feedback senden
- Titel
- Structural analysis of patterns and shapes using hierarchical vibrations
- Sprache
- Englisch
- Autor*innen
- Karin Engel
- Verlag
- Verl. Dr. Hut
- Erscheinungsdatum
- 2011
- Einband
- Hardcover
- ISBN10
- 3843900531
- ISBN13
- 9783843900539
- Kategorie
- Informatik & Programmierung
- Beschreibung
- The structural representation of shapes and pattern spaces is an important theme in computer vision and image analysis. For example, shape is one of the main sources of information that can be used for the classification and segmentation of images, as well as for multi-modal data fusion. Image segmentation can be seen as an ill-posed, inverse problem, which may have multiple possible solutions that do not continuously depend on the data. One reason for this is that images often come noisy and incomplete, while the texture and shape of visual objects may vary dramatically due to articulation, occlusion or variations in perspective and lightning conditions. A popular top-down approach to this problem is to collect a manually labelled training set of images from which the shape and texture variation in an object class can be learnt. Probabilistic graphical models require intensive training for separating valid from invalid non-linear variation. This may not always be possible. Further, determining a prior feature distribution that is both, informative and general, is not straightforward. A structural decomposition approach is presented for the model-based analysis of variable visual shapes and patterns in images without the need for training. The finite element shape decomposition principle has been extended to describe and match deformable visual objects at different, spatially resolved levels of abstraction and detail. A set of knowledge-based a-priori constraints in combination with general heuristics effectively condition the model to produce anatomically plausible shapes. The hierarchical shape decomposition approach offers a complete characterisation of the fitted shapes in terms of qualitative and quantitative features. The visual object model has been proven to represent various object categories. The a-priori constrained parametrisation allows interpolation between exemplar configurations, and accounts for “unknown” variations very well. We show experimentally that a valid parametric prior can be built from expert prior knowledge in a straightforward manner. The advantages of the hierarchical approach imply the training of specialised models from an abstract prototype and benefit of categorisation.