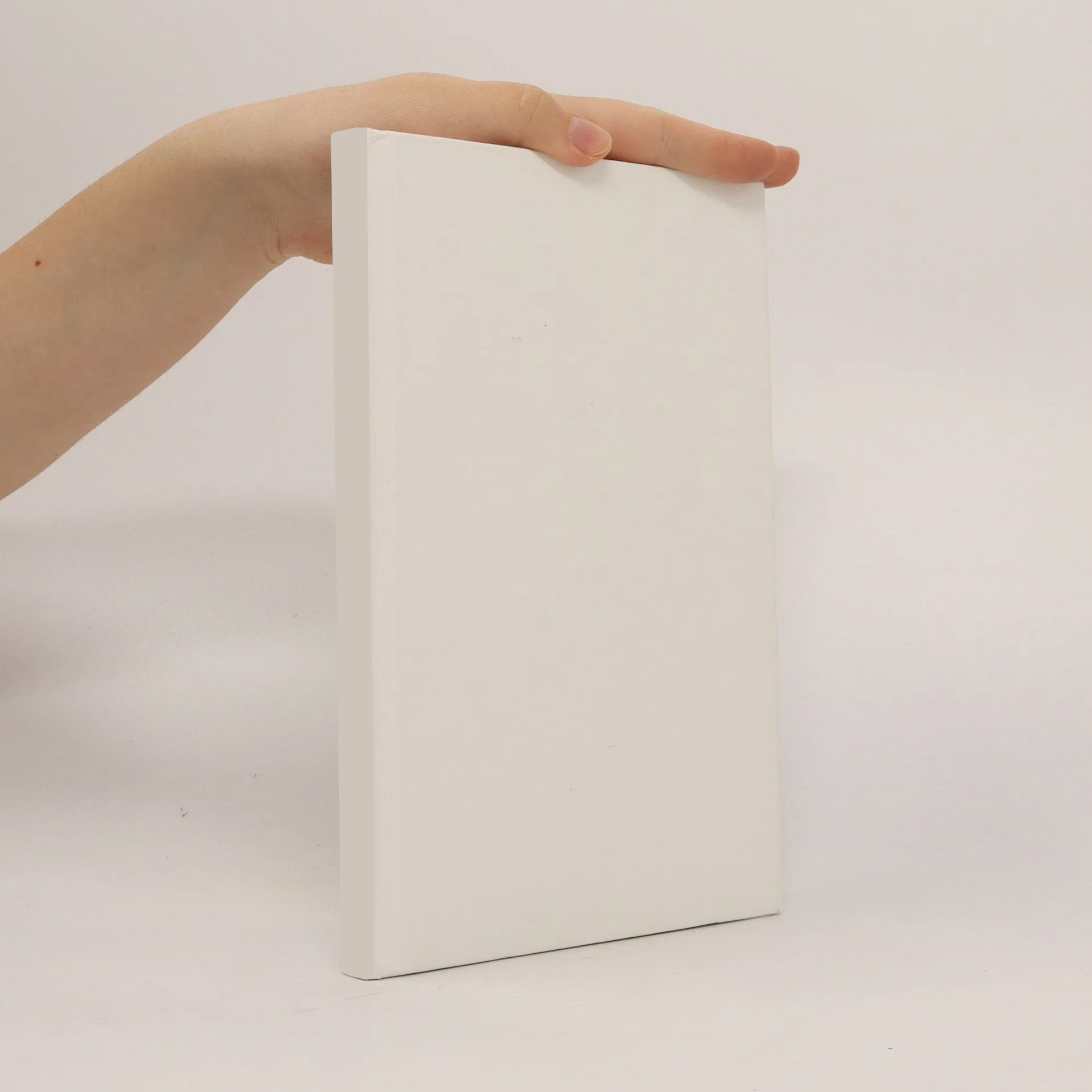
Tensor approximation methods for stochastic problems
Autoren
Parameter
Mehr zum Buch
Spectral stochastic methods have gained wide acceptance as a tool for efficient modelling of uncertain stochastic systems. The advantage of those methods is that they provide not only statistics, but give a direct representation of the measure of the solution as a so-called surrogate model, which can be used for very fast sampling. Especially attractive for elliptic stochastic partial differential equations (SPDEs) is the stochastic Galerkin method, since it preserves essential properties of the differential operator. One drawback of the method is, however, that it requires huge amounts of memory, as the solution is represented in a tensor product space of spatial and stochastic basis functions. Different approaches have been investigated to reduce the memory requirements, for example, model reduction techniques using subspace iterations to reduce the approximation space or methods of approximating the solution from successive rank-1 updates. In the present thesis best approximations to the solutions of linear elliptic SPDEs are constructed in low-rank tensor representations. By using tensor formats for all random quantities, the best subsets for representing the solution are computed “on the fly” during the entire process of solving the SPDE. As those representations require additional approximations during the solution process it is essential to control the convergence of the solution. Furthermore, special issues with preconditioning of the discrete system and stagnation of the iterative methods need adequate treatment. Since one goal of this work was practical usability, special emphasis has been given to implementation techniques and their description in the necessary detail.
Buchkauf
Tensor approximation methods for stochastic problems, Elmar K. Zander
- Sprache
- Erscheinungsdatum
- 2013
Lieferung
Zahlungsmethoden
Deine Änderungsvorschläge
- Titel
- Tensor approximation methods for stochastic problems
- Sprache
- Englisch
- Autor*innen
- Elmar K. Zander
- Verlag
- Shaker
- Erscheinungsdatum
- 2013
- ISBN10
- 3844018719
- ISBN13
- 9783844018714
- Reihe
- Berichte aus der Mathematik
- Kategorie
- Skripten & Universitätslehrbücher
- Beschreibung
- Spectral stochastic methods have gained wide acceptance as a tool for efficient modelling of uncertain stochastic systems. The advantage of those methods is that they provide not only statistics, but give a direct representation of the measure of the solution as a so-called surrogate model, which can be used for very fast sampling. Especially attractive for elliptic stochastic partial differential equations (SPDEs) is the stochastic Galerkin method, since it preserves essential properties of the differential operator. One drawback of the method is, however, that it requires huge amounts of memory, as the solution is represented in a tensor product space of spatial and stochastic basis functions. Different approaches have been investigated to reduce the memory requirements, for example, model reduction techniques using subspace iterations to reduce the approximation space or methods of approximating the solution from successive rank-1 updates. In the present thesis best approximations to the solutions of linear elliptic SPDEs are constructed in low-rank tensor representations. By using tensor formats for all random quantities, the best subsets for representing the solution are computed “on the fly” during the entire process of solving the SPDE. As those representations require additional approximations during the solution process it is essential to control the convergence of the solution. Furthermore, special issues with preconditioning of the discrete system and stagnation of the iterative methods need adequate treatment. Since one goal of this work was practical usability, special emphasis has been given to implementation techniques and their description in the necessary detail.