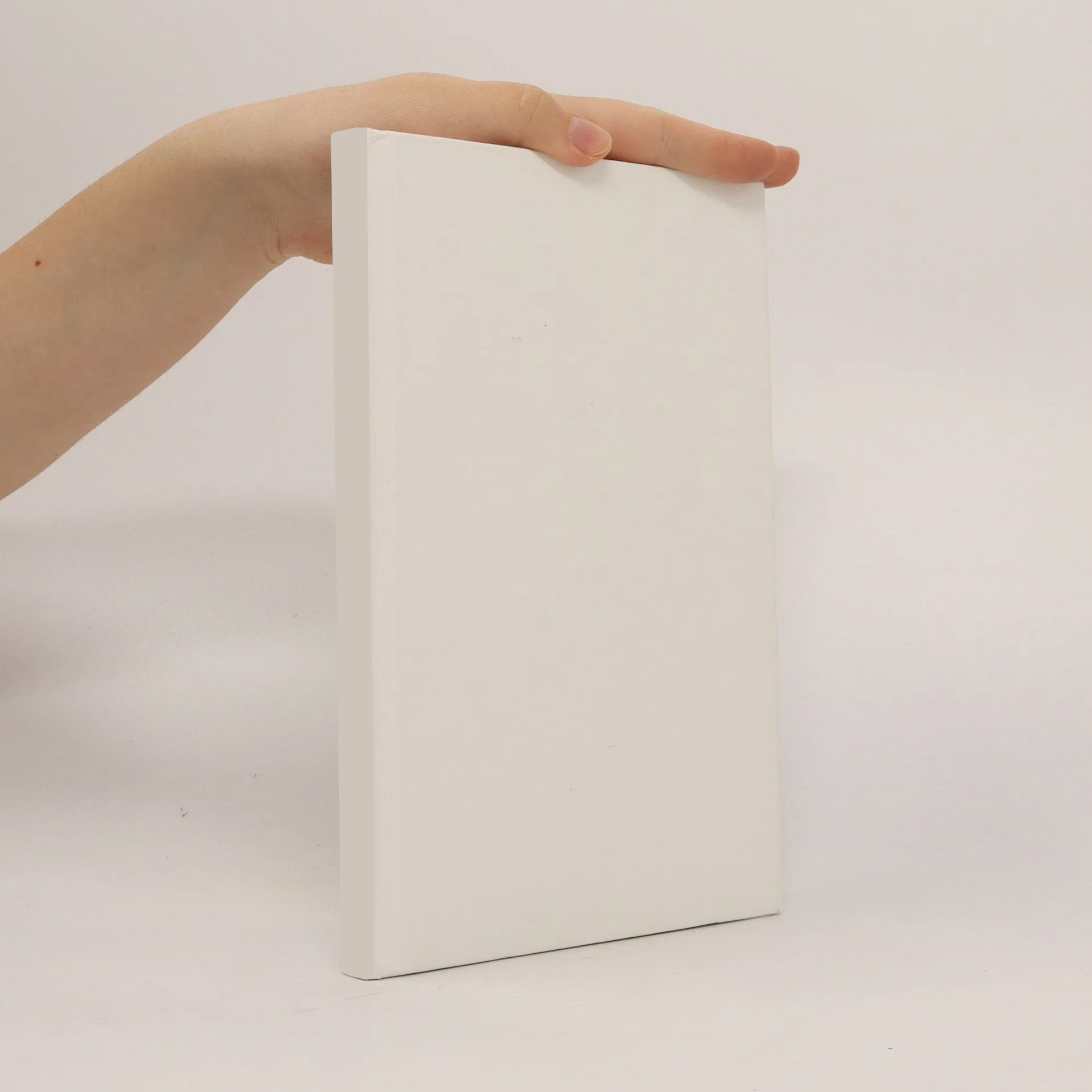
Decision making in financial markets
Autoren
Mehr zum Buch
This work extends the discussion on artificial markets research using a novel approach to model decision making in financial markets. The topic is motivated through a thorough overview on economic and behavioral decision making as well as a review of the stock market simulation literature. A decision making model including various behavioral components is thereafter presented and the model is validated utilizing a multi-agent-multi-period stock market simulation. The simulation utilizes a genetic algorithm as proxy for individual learning and a cellular omaton as proxy for social learning, behavioral aspects such as changing risk-aversion, overconfidence, and the disposition effect are implemented using a particular utility function. During the simulation agents learn to forecast a price from market signals - including variables such as the fundamental value, charting information, news, and social learning - and generate periodic trading decisions. The trading decisions are aggregated and in a market clearing process a market price emerges. The resulting time series is analyzed and compared to the statistical properties of a random walk as well as to market data. Results indicate that simulation runs with agents parameterized to represent rational investors generate time series that exhibit the properties of a random walk, for parameterizations including behavioral aspects the simulated data replicates various stylized facts known to exist for market data, such as fat tailed distributions of returns, long memory effects, and the Taylor effect.