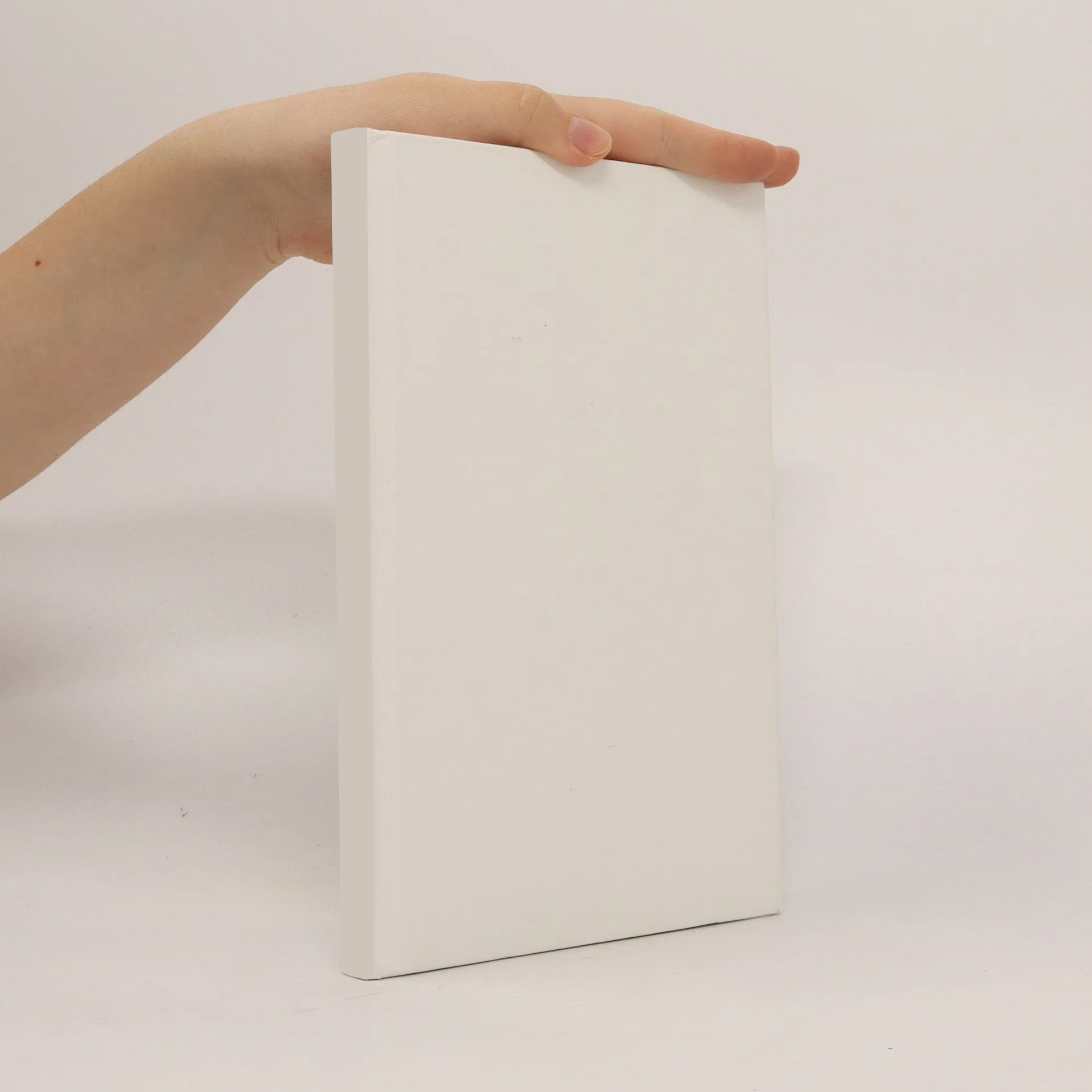
Aerodynamic optimization of low-pressure axial fans
Autoren
Parameter
Mehr zum Buch
This Ph. D. Thesis discusses the aerodynamic optimization of low pressure axial fans. Three major issues are discussed: 1. What is the upper limit of total-to-static efficiency that is achievable with axial fans and how do geometrical constraints compromise this limit? 2. Which impeller geometry is required to obtain maximum efficiency? 3. How significant is the improvement over fans designed with state-of-the art methods and what is the aerodynamic explanation for the improvement? All of these questions are discussed in the context of various design points. The key element of the methodology is an evolutionary optimization strategy in which the fan performance is predicted by CFD-trained artificial neural networks. The methodology is successfully validated by experimental tests with prototypes.
Buchkauf
Aerodynamic optimization of low-pressure axial fans, Konrad Bamberger
- Sprache
- Erscheinungsdatum
- 2015
Lieferung
Zahlungsmethoden
Deine Änderungsvorschläge
- Titel
- Aerodynamic optimization of low-pressure axial fans
- Sprache
- Englisch
- Autor*innen
- Konrad Bamberger
- Verlag
- Shaker Verlag
- Erscheinungsdatum
- 2015
- ISBN10
- 3844040315
- ISBN13
- 9783844040319
- Reihe
- Berichte aus der Strömungstechnik
- Kategorie
- Skripten & Universitätslehrbücher
- Beschreibung
- This Ph. D. Thesis discusses the aerodynamic optimization of low pressure axial fans. Three major issues are discussed: 1. What is the upper limit of total-to-static efficiency that is achievable with axial fans and how do geometrical constraints compromise this limit? 2. Which impeller geometry is required to obtain maximum efficiency? 3. How significant is the improvement over fans designed with state-of-the art methods and what is the aerodynamic explanation for the improvement? All of these questions are discussed in the context of various design points. The key element of the methodology is an evolutionary optimization strategy in which the fan performance is predicted by CFD-trained artificial neural networks. The methodology is successfully validated by experimental tests with prototypes.