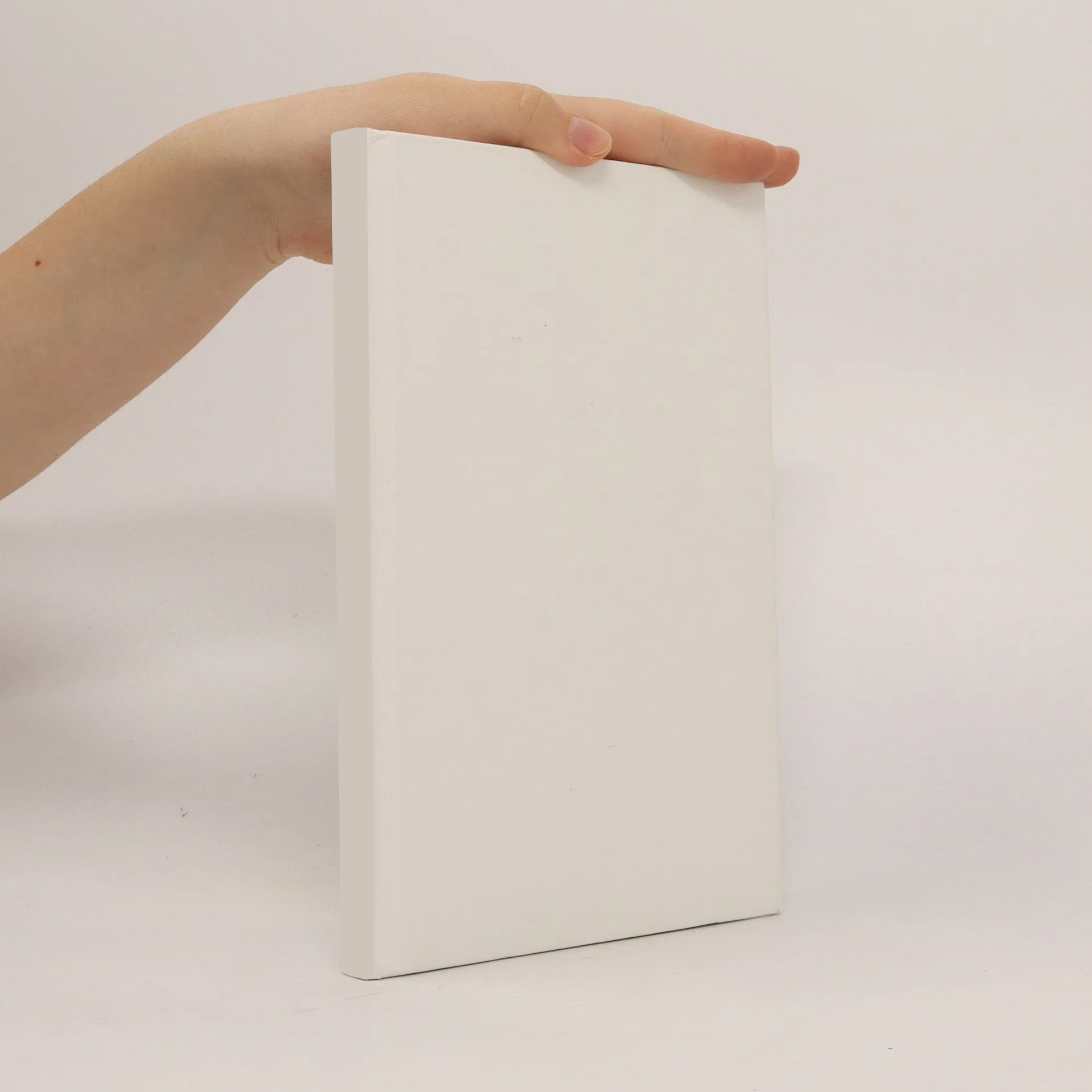
Deconvolution of industrial measurement and manufacturing processes for improved process capability assessments
Autoren
Parameter
Mehr zum Buch
The accurate estimation of process capability indices is essential for manufacturers, since the dimensional and geometric accuracy of individual parts is necessary, to guarantee the functionality of the product and to ensure the interchangeability of components. In the presence of measurement uncertainty, the current technique for estimating the value of the process capability index, Cp, systematically underestimates the Cp value and can even result in grossly inaccurate statements about the uncertainty attributed to the estimation. The objective of this research is to develop an approach for evaluating the process capability of a normally distributed manufacturing process, which reduces the bias caused by the uncertainty of individual sample measurements and provides accurate uncertainty estimations for the resulting Cp value. The problem is approached by applying a statistical technique called deconvolution to mathematically compensate for the known measurement uncertainty and to derive a new estimator for the Cp index. Furthermore, the current measurement model for Cp estimations is extended to account for the uncertainty of sample measurements in confidence interval calculations. The results are experimentally validated via Monte Carlo simulation and compared to the current estimation method. It is concluded that for sufficiently large sample sizes, the proposed deconvolution approach successfully reduces the bias caused by the uncertainty of individual sample measurements and accurately states the uncertainty associated with the estimation. The method finds its primary application in the manufacturing of microstructures, where the uncertainty of measurement can be of a similar magnitude to the variation of the manufacturing process.
Buchkauf
Deconvolution of industrial measurement and manufacturing processes for improved process capability assessments, Kyle James Kippenbrock
- Sprache
- Erscheinungsdatum
- 2018
Lieferung
Zahlungsmethoden
Deine Änderungsvorschläge
- Titel
- Deconvolution of industrial measurement and manufacturing processes for improved process capability assessments
- Sprache
- Englisch
- Autor*innen
- Kyle James Kippenbrock
- Verlag
- Shaker Verlag
- Erscheinungsdatum
- 2018
- ISBN10
- 3844061401
- ISBN13
- 9783844061406
- Reihe
- Forschungsberichte aus dem wbk, Institut für Produktionstechnik, Karlsruher Institut für Technologie (KIT)
- Kategorie
- Skripten & Universitätslehrbücher
- Beschreibung
- The accurate estimation of process capability indices is essential for manufacturers, since the dimensional and geometric accuracy of individual parts is necessary, to guarantee the functionality of the product and to ensure the interchangeability of components. In the presence of measurement uncertainty, the current technique for estimating the value of the process capability index, Cp, systematically underestimates the Cp value and can even result in grossly inaccurate statements about the uncertainty attributed to the estimation. The objective of this research is to develop an approach for evaluating the process capability of a normally distributed manufacturing process, which reduces the bias caused by the uncertainty of individual sample measurements and provides accurate uncertainty estimations for the resulting Cp value. The problem is approached by applying a statistical technique called deconvolution to mathematically compensate for the known measurement uncertainty and to derive a new estimator for the Cp index. Furthermore, the current measurement model for Cp estimations is extended to account for the uncertainty of sample measurements in confidence interval calculations. The results are experimentally validated via Monte Carlo simulation and compared to the current estimation method. It is concluded that for sufficiently large sample sizes, the proposed deconvolution approach successfully reduces the bias caused by the uncertainty of individual sample measurements and accurately states the uncertainty associated with the estimation. The method finds its primary application in the manufacturing of microstructures, where the uncertainty of measurement can be of a similar magnitude to the variation of the manufacturing process.