Das Buch ist derzeit nicht auf Lager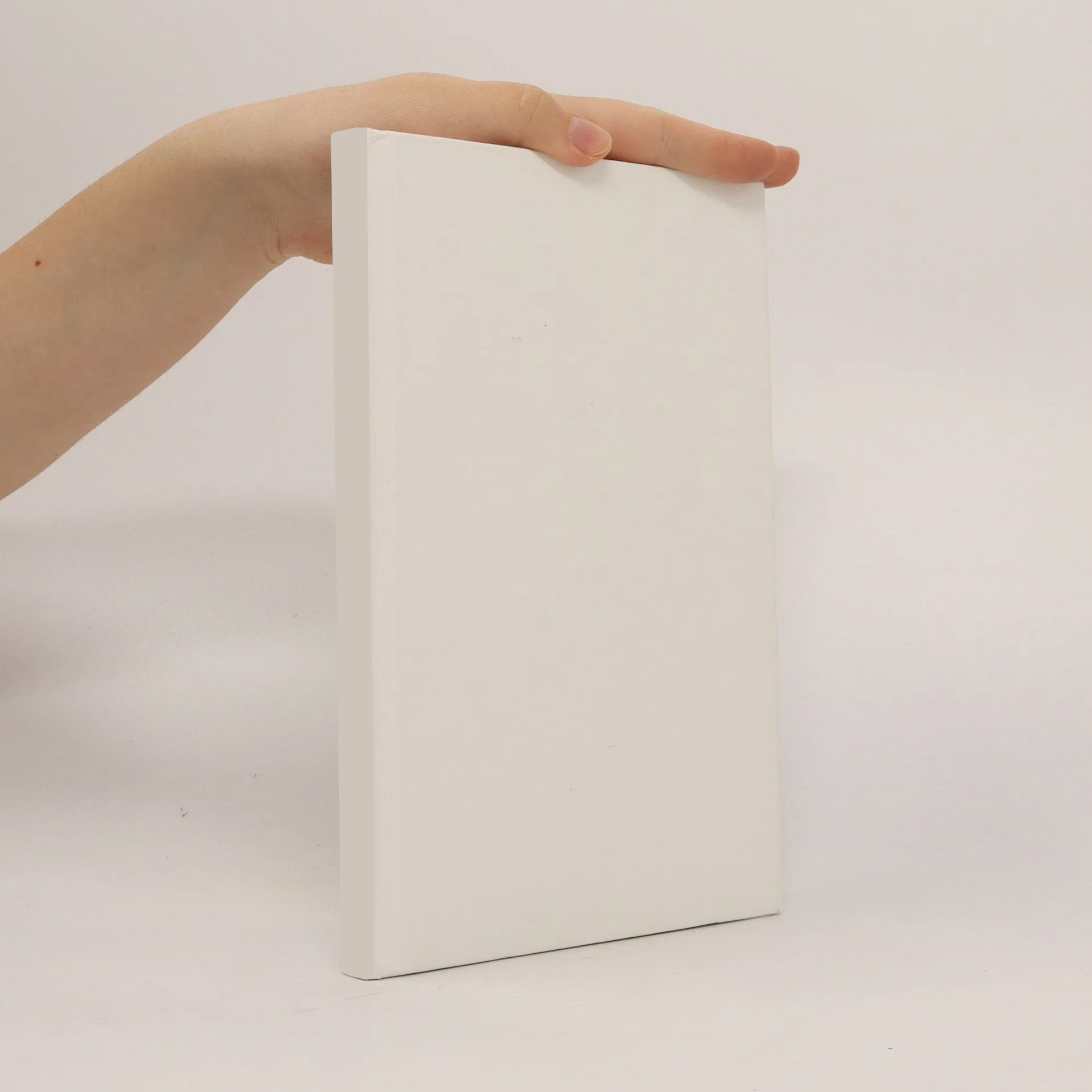
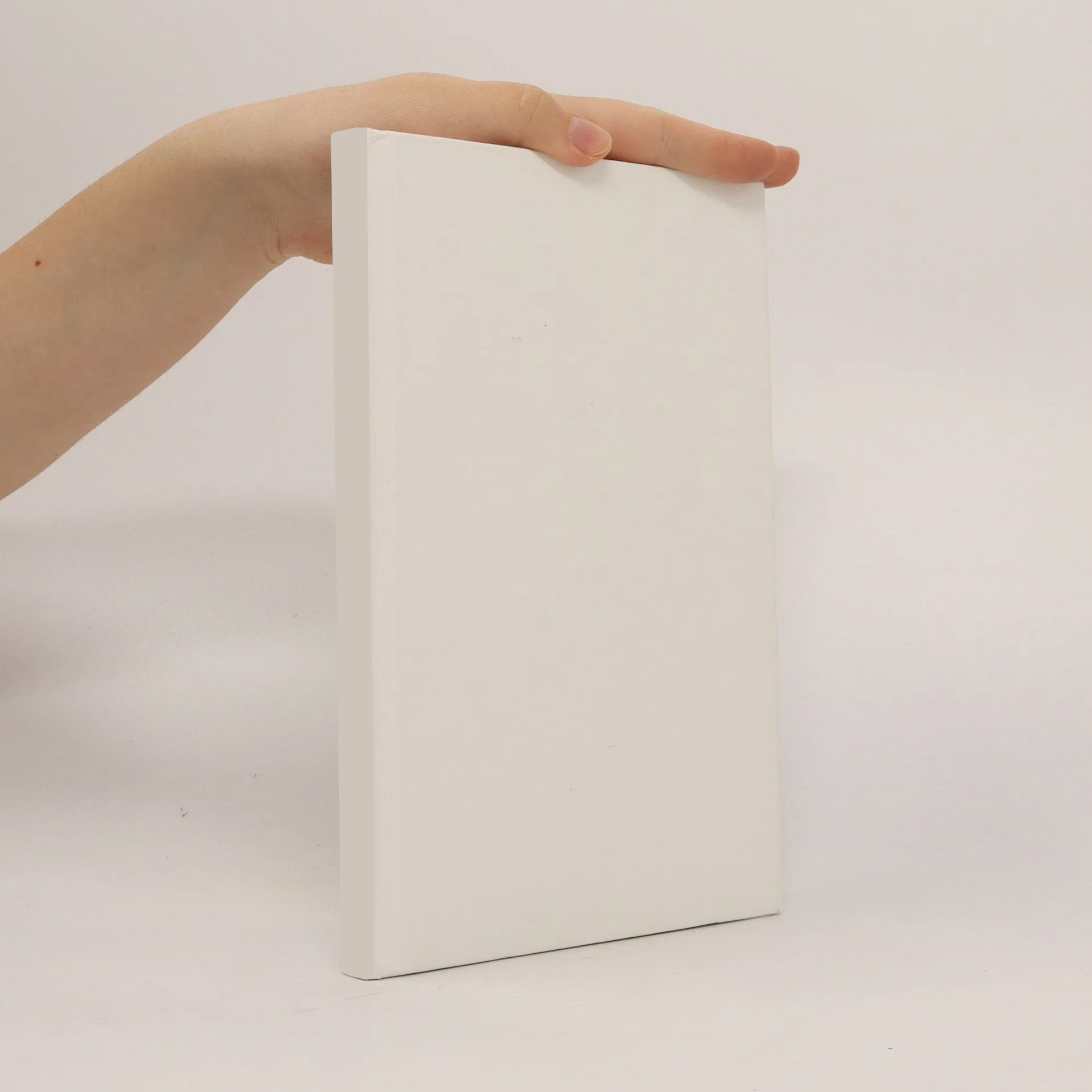
Hierarchical Relative Entropy Policy Search
An Information Theoretic Learning Algorithm in Multimodal Solution Spaces for Real Robots
Autoren
68 Seiten
Mehr zum Buch
The book explores the significance of hierarchical structures in enhancing scalability and performance in motor skill tasks. It introduces the concept of a "mixed option policy," where a gating network selects which option to execute, followed by an option-policy that determines the action. This hierarchical approach enables the learning of multiple solutions to problems. The algorithm is grounded in an innovative information theoretic policy search method that effectively manages the exploitation-exploration trade-off, minimizing information loss during policy updates.
Buchvariante
Buchkauf
Wir benachrichtigen dich per E-Mail.